Understand
Platformable's Data Governance model
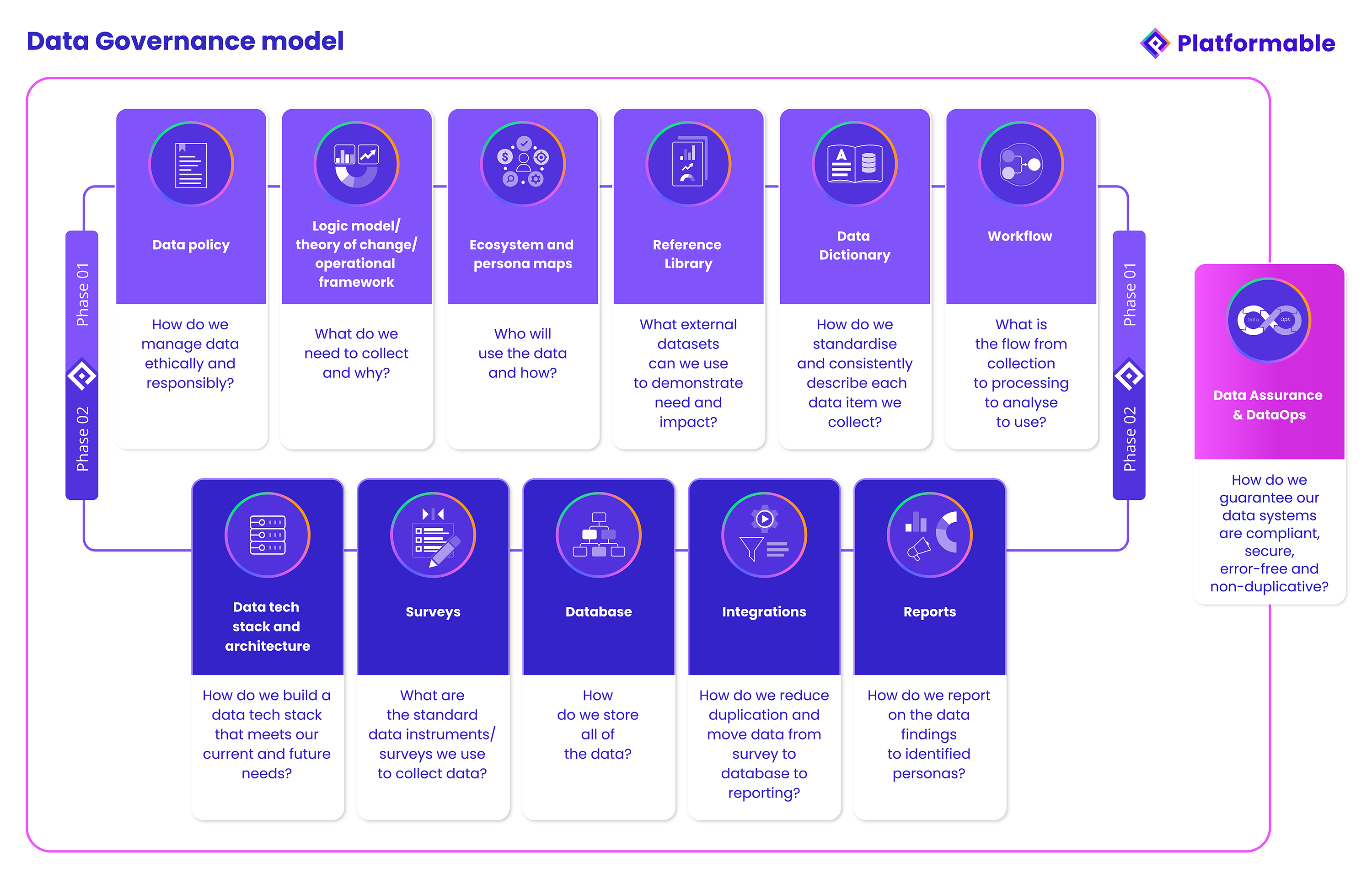
Data governance is fast becoming a core skill needed in any organisation that is working within a digital ecosystem. With the global move to digital infrastructures, every process, interaction, product, service, and outcome can be measured digitally, creating vast troves of data that can then be analysed and used to:
- Strategise and plan resources
- Map partner and end user needs
- Identify and address shortcomings and failures within a system, and
- Automate processes and workflows.
Data can also be packaged in a way that can be sold or shared, as we do at Platformable with datasets on open banking and open finance and on the API industry. And now, organisations are beginning to use their data (if it is well managed and governed) as the source for large language models and other machine learning processes that create artificial intelligence solutions.
At Platformable, we have long been involved in data governance processes:
- As founder/director, I have been involved in data governance systems throughout my career, including using data to advocate for the rights of people with HIV/AIDS during the early days of the HIV/AIDS epidemic in Australia, and in developing data models for local governments (including data dictionaries and data-driven decision making processes) to address health and well-being needs of local populations
- At Platformable, we have developed data governance systems for equity-focused health non-profits, including mentoring of key staff, development of data teams, and creation of apps that support organisations to manage all of their data
- Consulting for the Open Data Institute in the UK , we have written a data governance playbook for Roche, assessed organisation's data governance capacities in low and middle income countries, and worked with the World Health Organisation on a global review of data governance maturity in the health sector
- We have developed data governance processes and supported data governance initiatives of private clients in multiple sectors including intellectual property, sustainability, and privacy technology.
Throughout these activities, we have built on our processes in order to create a Data Governance model that can help any organisation to manage their data at all stages of the data journey.
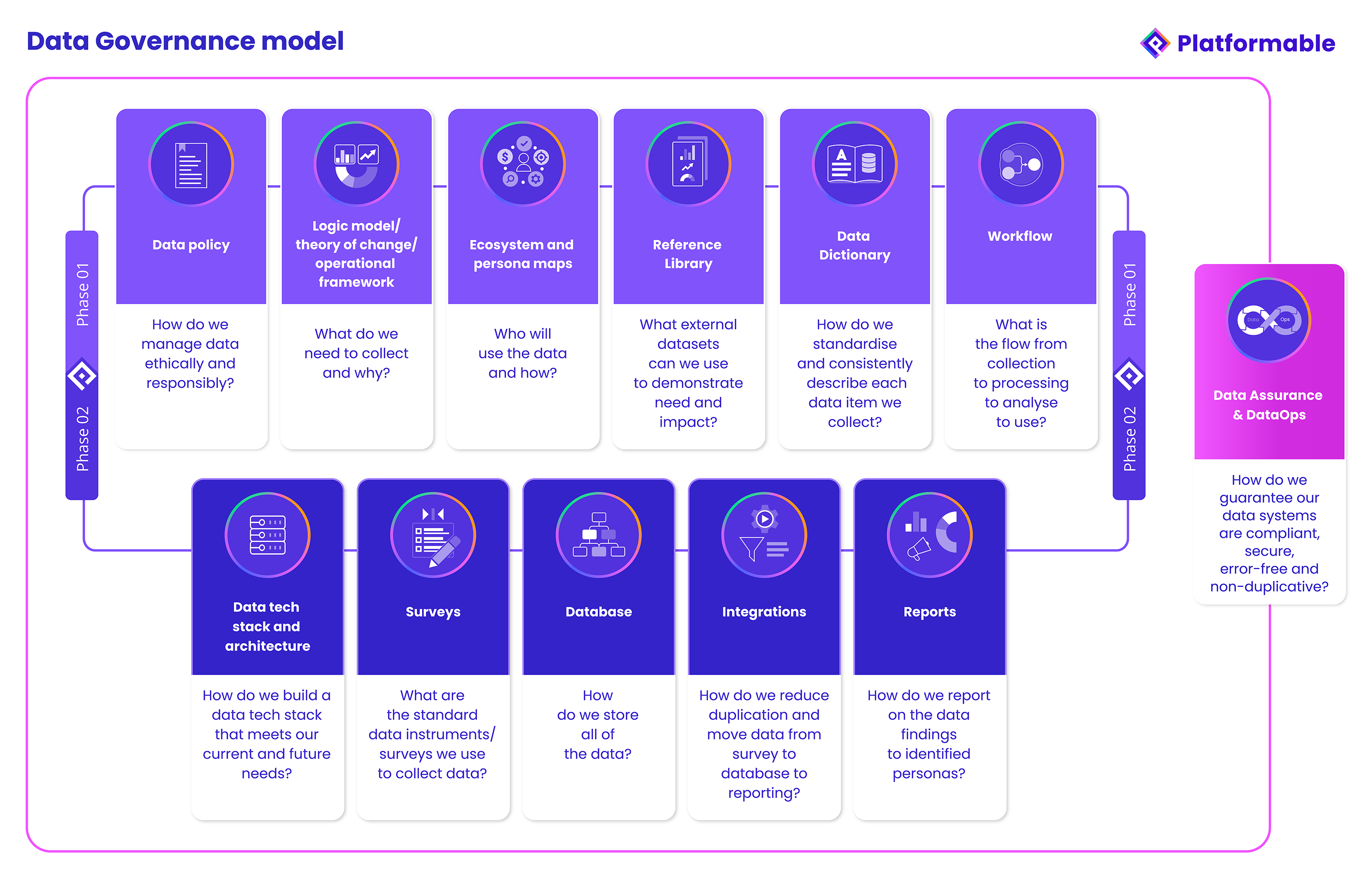
The Data Journey refers to how data is created, collected, transformed, stored, managed, analysed and potentially shared.
While our Data Governance model can be implemented in a linear fashion, we also find that many organisations have already commenced some work in these areas. This model can then be used as a maturity scorecard which can highlight areas might need further work in order to create a more comprehensive approach to data management.
Data Governance Phase 1: Laying the foundation
We see there are two phases of implementation needed in a data governance model.
Phase 1 refers to the decisions and foundational elements that make it possible to manage data in a standardised, ethical, responsible manner. During Phase 1, a data manager or data lead may work across an organisation to build out a library of components that will help make consistent data journey decisions and automation possible in the future.
Phase 1 involves 6 steps. These can be conducted in any order, or can be created as a basic framework at first, and then further developed as the organisation becomes more mature around harnessing the value of data.
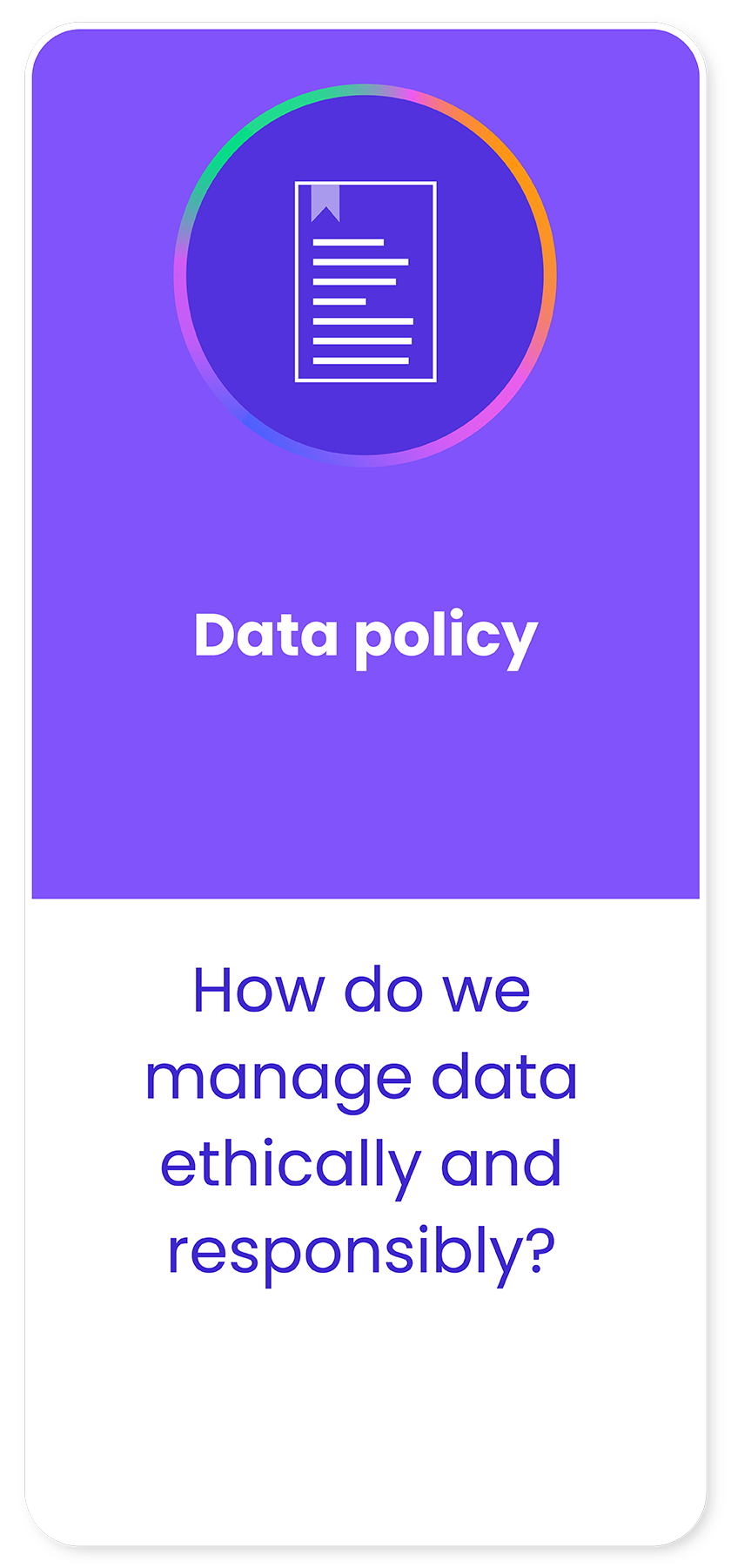
Data policy: A data policy outlines the "rules of engagement" for an organisation's use of data. Data ethics processes may be conducted to assist in defining data policies, processes to discuss how data will be collected and stored may be documented, and so on. A data policy answers the question: "How do we manage data ethically and responsibly?" When an organisation is collecting data from an individual or from an organisation, they may be asked for proof that their data will be respected and managed appropriately. A data policy is the evidence that shows the organisation is ready to take data use seriously.
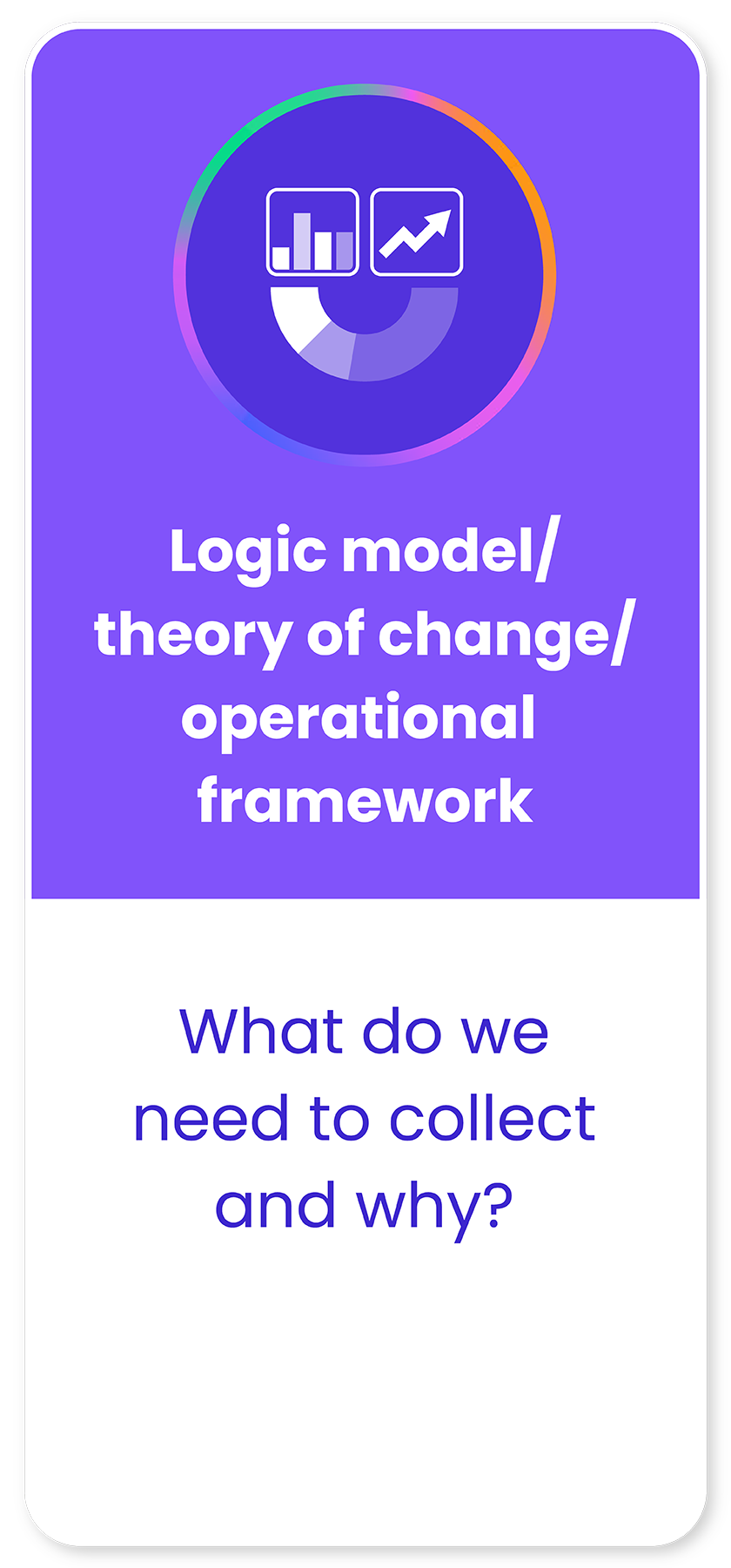
Logic model/theory of change/operational framework: There are multiple data points that are collected or used during any process, in any sector. It is useful to document a theory of change or logic model that describes an organisation's reason for existence, processes, product flow, or so on. For each of these organisational aspects, when we map the theory of change or logic model which describes how the organisation operates or intends to achieve impacts and outcomes, we can more readily identify what data can or needs to be collected along the way. For non-government organisations we have worked with, for example, they are often funded by specific government departments to achieve particular goals or outcomes. By mapping their funded program logic model, they can show how their actions are expected to impact on the communities they serve. This helps them identify what data they need to collect in terms of outputs and outcomes.
For example, at Platformable, we have developed open ecosystem value models that describe how value is generated and distributed in a general open ecosystem, and more specifically in health and open banking/open finance ecosystems. These value models help us identify what data needs to be collected and how to analyse this data to show that an ecosystem is generating the value expected for all stakeholders, and that value is being distributed across the ecosystem rather than being locked in by specific stakeholders, creating power imbalances. These models help us answer the question: "What data do we need to collect and why?"
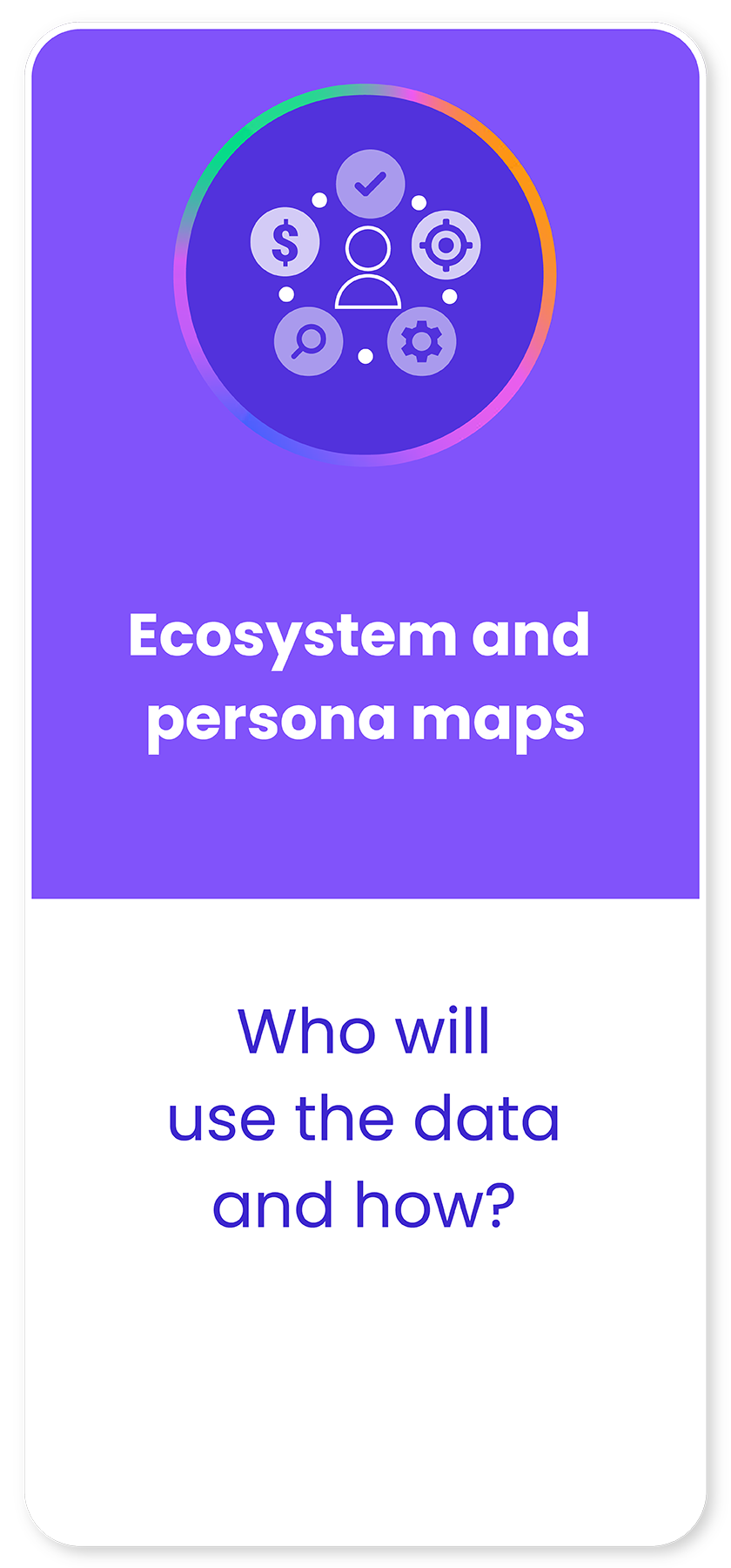
Ecosystem and persona maps: Understanding where data can be sourced from, how it can be combined with other data sets, who needs to use it and why, assists data governance by ensuring that data collected is fit-for-purpose, and that it can be transformed/standardised and used in comparison with other datasets. Mapping the data ecosystem and data user personas also helps guide data governance decisions around storage and data sharing agreement preparations. This stage of the process asks "Who will use the data and how?"
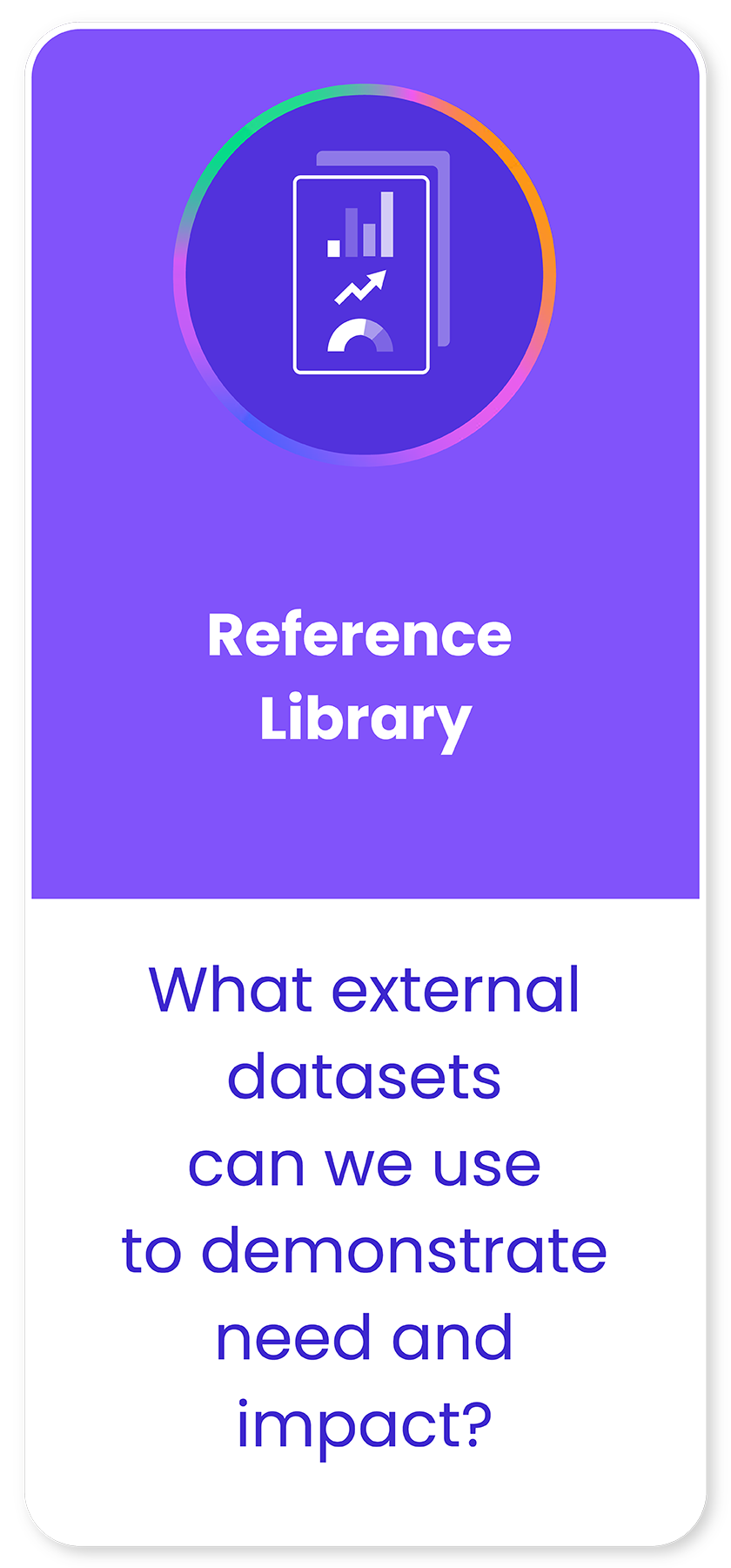
Reference Library: Wherever possible, it is best to collect data using standardised data models and data standards. This will allow data to be compared with other datasets. If data is being sold or shared, it allows the data to be more valuable to external users by being immediately understandable and interoperable. Sometimes there are not data model standards per se being used by industry sectors or in specific fields, but there are specific large datasets that are used for industry-wide or population-wide analysis. Where this is the case, it is often worth understanding the data dictionaries and methodologies of those datasets and ensuring that your own dataset follows the same methodology (in that way, you can use your data to compare your impacts with the outcomes for wider populations or across an industry). Creating a reference library of data standards, data models, and existing datasets that might be used to compare with your own data sets helps guide you in how to collect your own data, and helps you understand how your data collection might be used to compare with wider datasets. This stage asks "What external datasets can we use to demonstrate need and impact?"
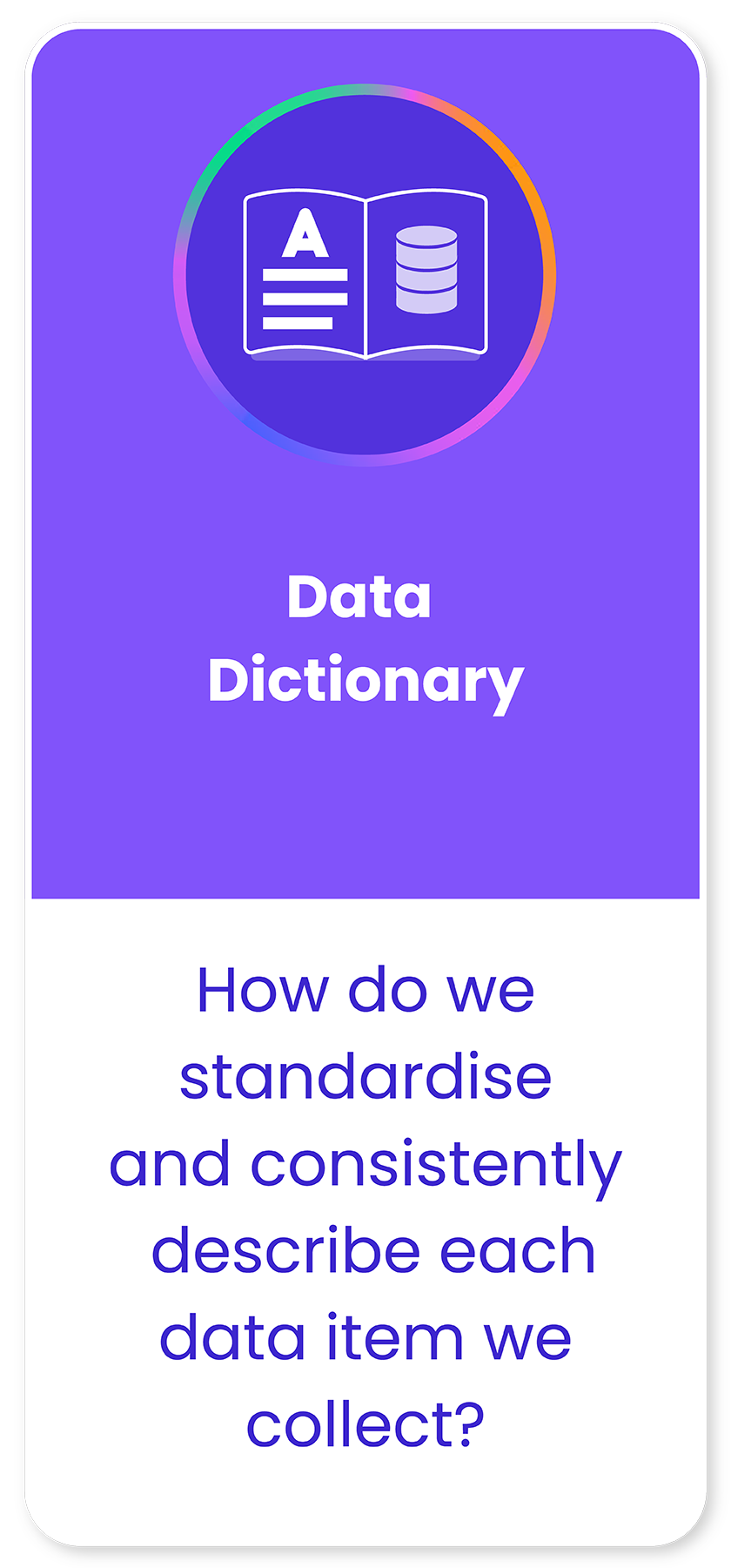
Data dictionary: It is essential that all datasets are fully described. This helps collectors and analysts catalogue data in the same way as everyone is using the same definition for data, but a data dictionary can also stipulate the data field type (date time format, integer, text, and so on), as well as set any validation rules (for example, that dates are always in the format DD/MM/YYYY). An organisation's data dictionary should be the first port of call when deciding on a new dataset: wherever possible, the organisation should use the standard formats used in the dictionary. For example, if a new dataset requires age to be collected, the data dictionary should be consulted and the way age is asked should be used (whether it be by age in years, date of birth, year of birth, or age range) so that the data collected is consistent with other data the organisation collects (there are some caveats here: if your data policy stipulates that you will take a data minimisation approach to managing data, the organisation may collect year of birth or age range for the majority of datasets where age is needed for people's data, but for something like financial identification, loyalty programs, or clinical services it may be necessary to collect actual date of birth. The data dictionary can define when general age data is warranted and when more specific date of birth needs to be collected). This stage of data governance asks "How do we standardise and consistently describe each data item we collect?"
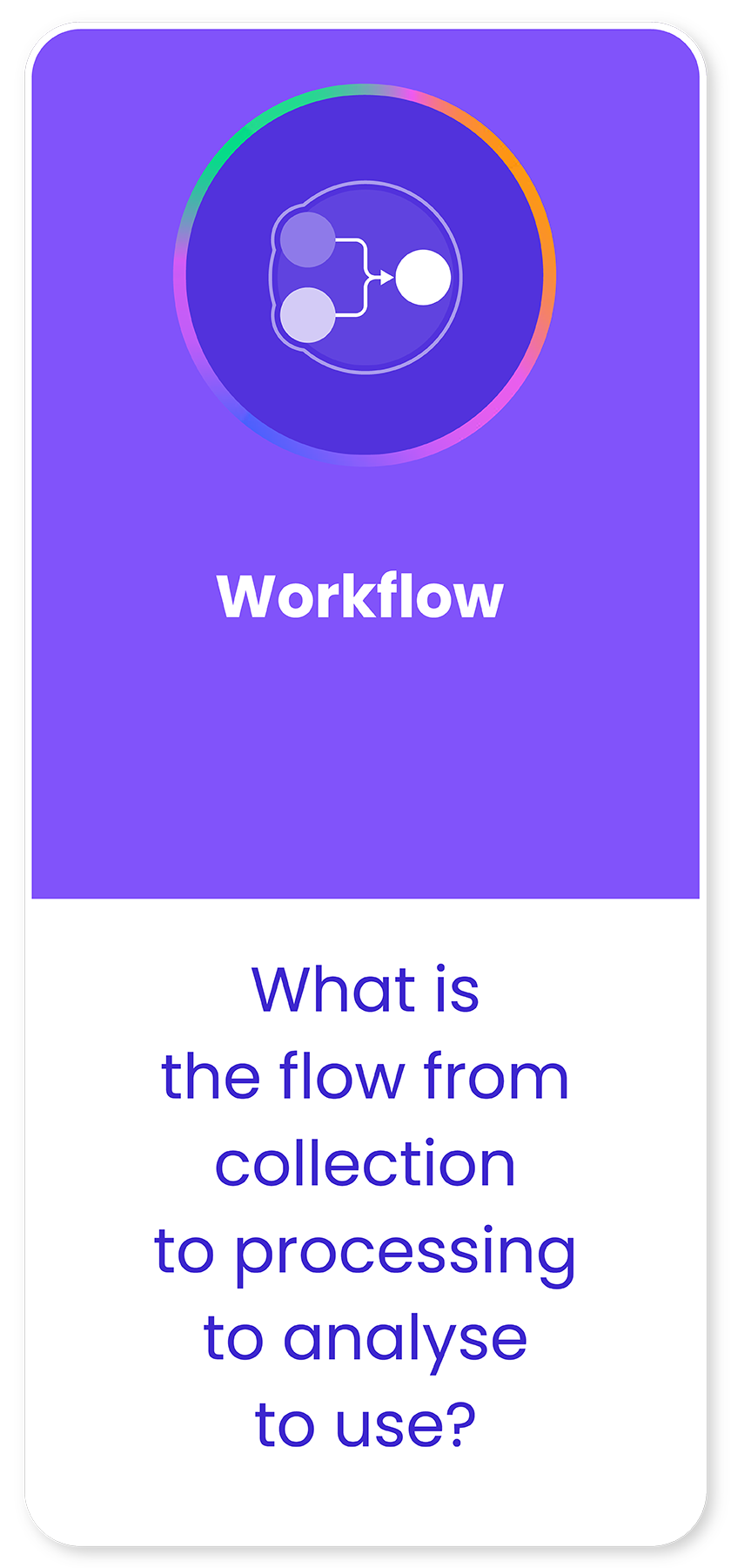
Workflow: It is useful now to map key processes within your organisation that reflect the data journey. These may be done in a 'user story' type approach, using flow diagrams to describe key data management tasks. For example, there may be workflows created for:
- Designing a new dataset or survey (including any user consent flow),
- Collecting and storing data (and how its is transformed and normalised),
- Accessing stored data securely to analyse or create visualisations,
- Generating reports, and
- Accessing data from a third party and/or sharing an organisation's data with an external partner or via a marketplace.
Mapping each of these flows will show when the data policy is drawn on, how external data sets (the reference library) and internal data dictionary (standards) are consulted, and so on. This is an important step before Phase 2, when automations are put in place to ensure the flow runs automatically without the need to allocate time to manually move data from one part of the data journey to the next. At this stage, we ask "What is the flow from collection to processing to analyse to use?"
Phase 1 focuses on all of the decisions and processes that need to be considered to best manage data across the data journey.
Data Governance Phase 2: Implementing an automated system
Phase 2 is now about implementing and automating these processes so that data can be managed more easily, freeing time for data usage, analysis, and data-sharing partnership development.
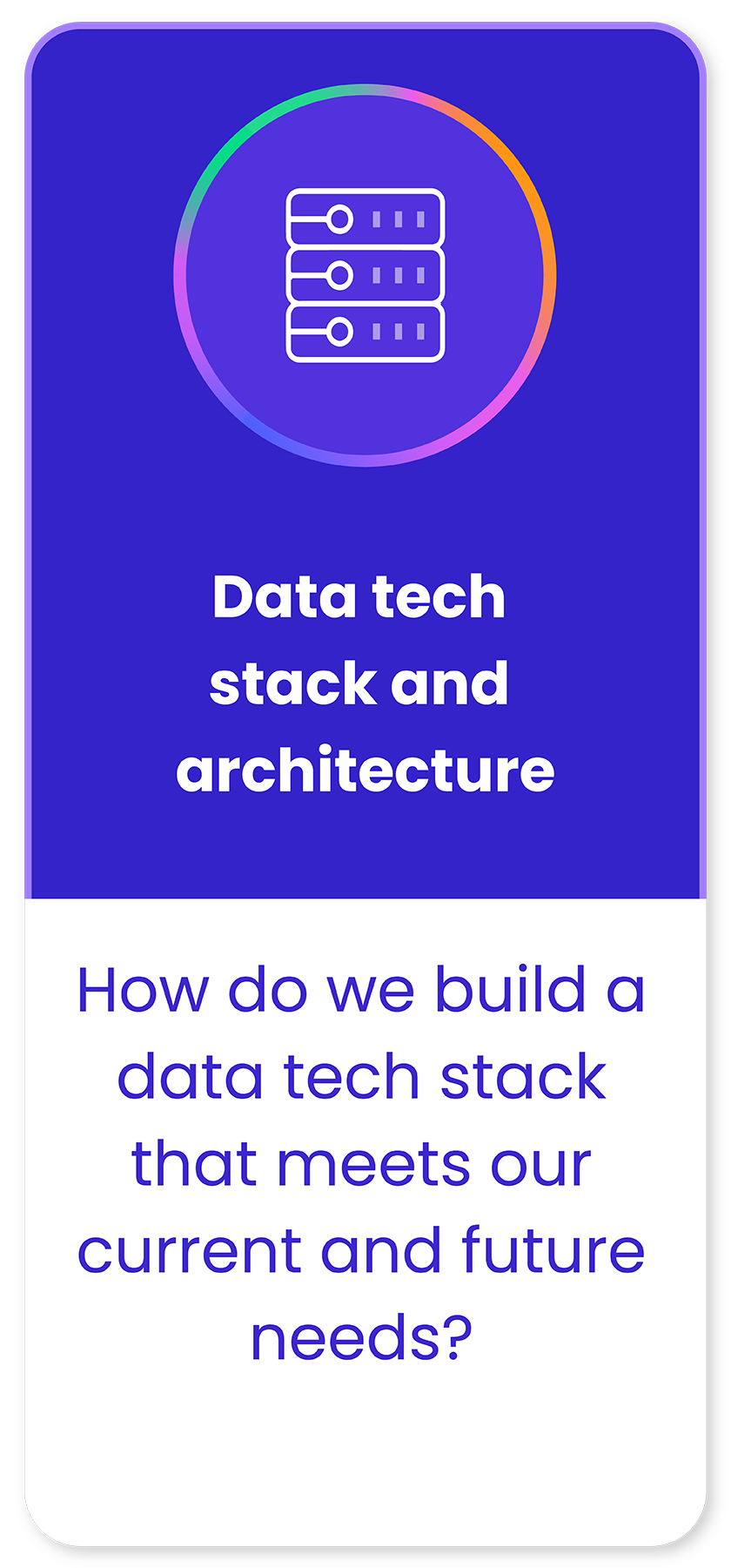
Data tech stack and architecture: Now that you understand all of the components involved in managing a comprehensive data governance framework, it is up to you to decide what technologies will help support you across the data journey. Some non-profits and smaller organisations use a combination of tools, like SurveyMonkey or Qualtrics for survey and data collection, Google Sheets for analysis on spreadsheets and creation of charts and graphics, Dropbox to store data, and tools like Zapier to automate moving data between various stages. Larger corporations might use BI tools and dashboards for analysis, connected to a data lake or data warehouse like Snowflake, and some off-the-shelf data governance software like Atlan that helps manage data dictionaries (for the main part). Others map out their data architecture and needs and have internal or contracted developer teams build an enterprise app specifically for them. The first stage of Phase 2 asks: "How do we build or buy a data tech stack that meets our current and future needs?"
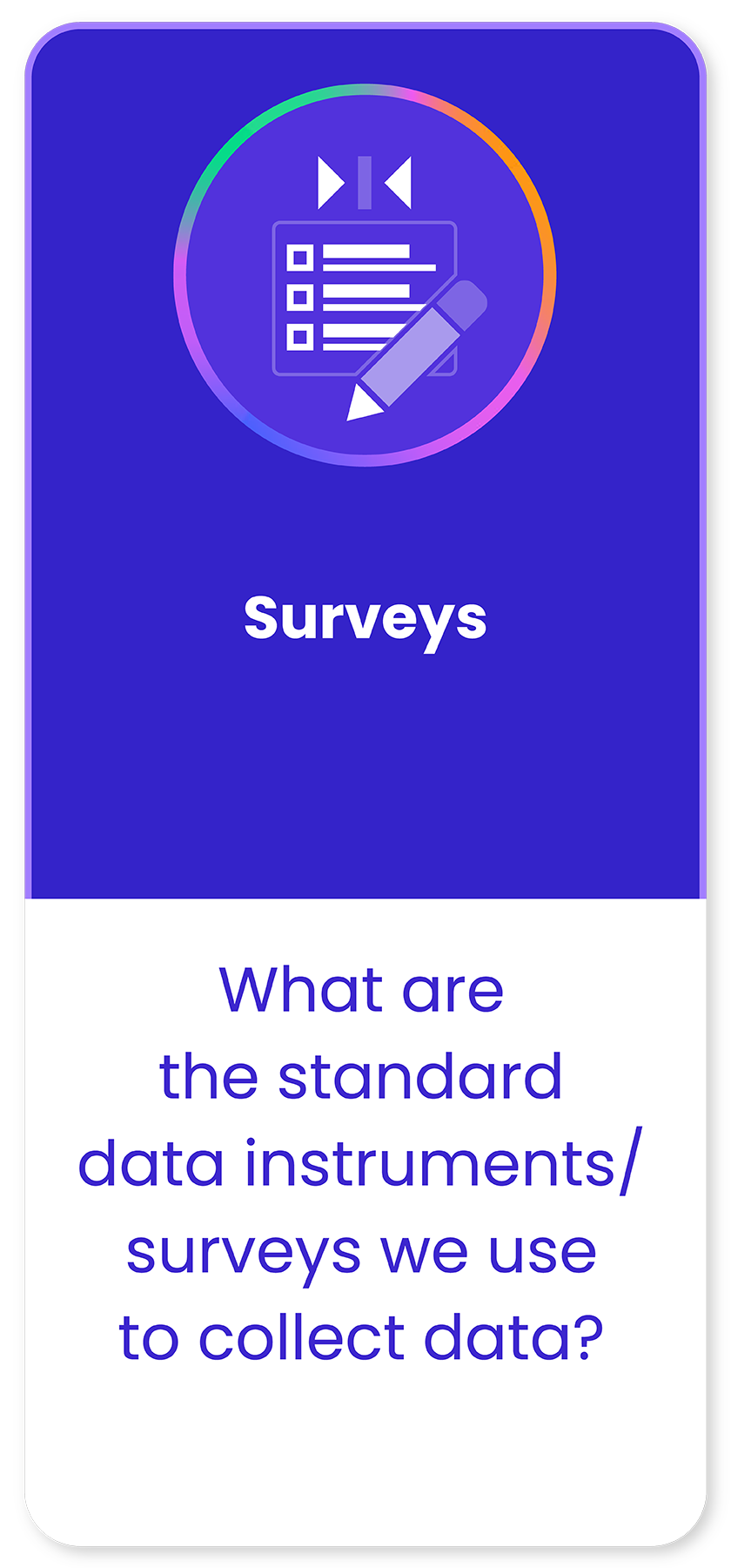
Surveys: With processes and components like data dictionaries in place, and an understanding of wider datasets catalogued in our reference library, along with understanding what data we need to collect from our program logic model or theory of change, we are now ready to create the data collection instruments, usually in the form of surveys and forms. We consult with the data dictionary to make sure we are using standardised question and answer formats, and we may do another logic model if the survey is for a specific program or new use case, to double check that we are collecting the data we will need to report and analyse later on. We ask "What are the standard data instruments/surveys we use to collect data?"
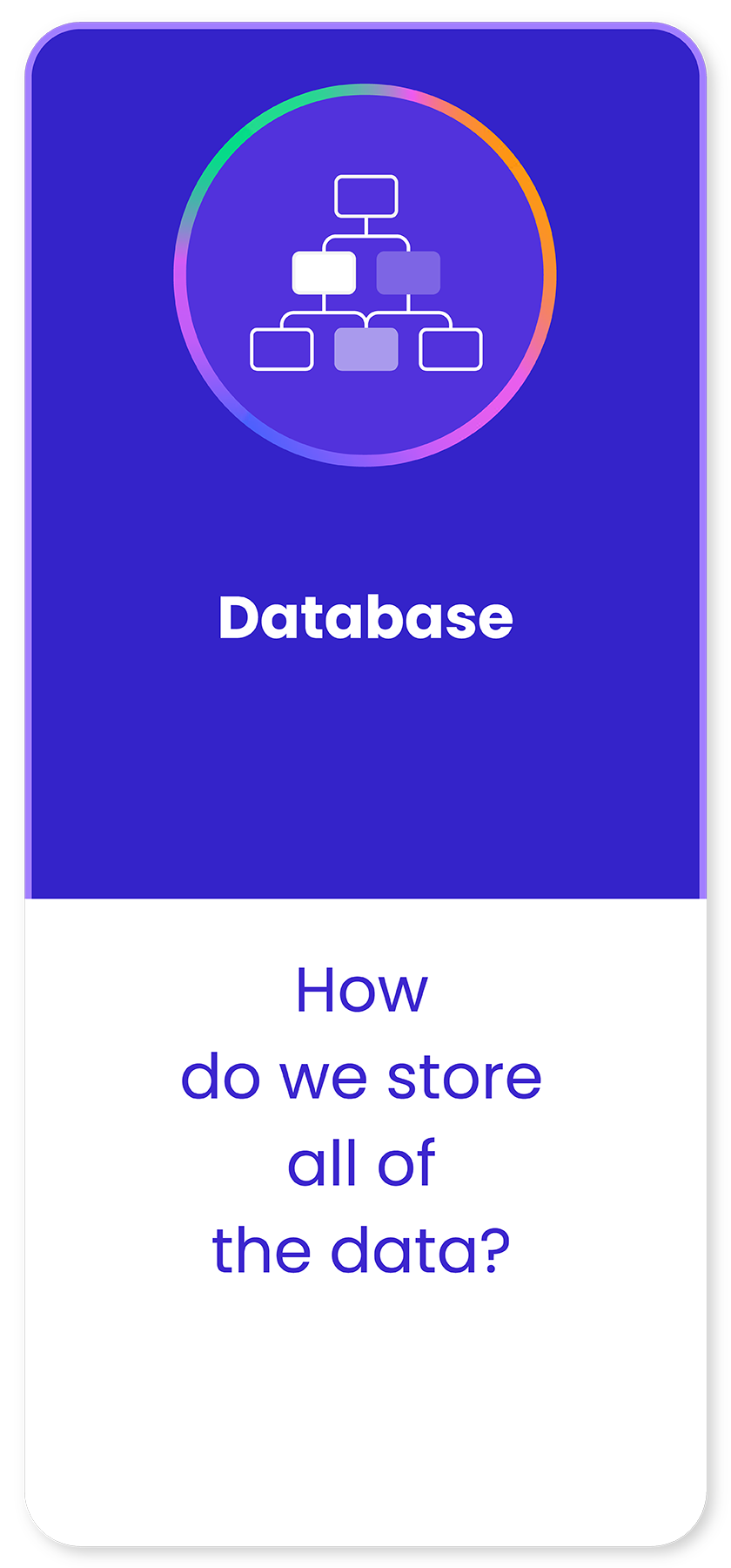
Database: We now start to build the database. In reality, we may jump back and forth between survey design and database design. We find that designing the data models that will inform the database is more easily done when we understand the data collection process. In any case, this stage draws on many of the components in stage 1 to help inform the data model design and when the data model is defined, a database is created with the necessary tables. Again, the data tech stack will help identify if data modelling tools that automatically generate the databases will be used (such as DBSchema), or whether the survey builder software or other tools already have a database backend that gets created, and so on. In those cases, this stage is more about customisation and ensuring validation rules of data that match the agreed decisions documented in the data dictionary.
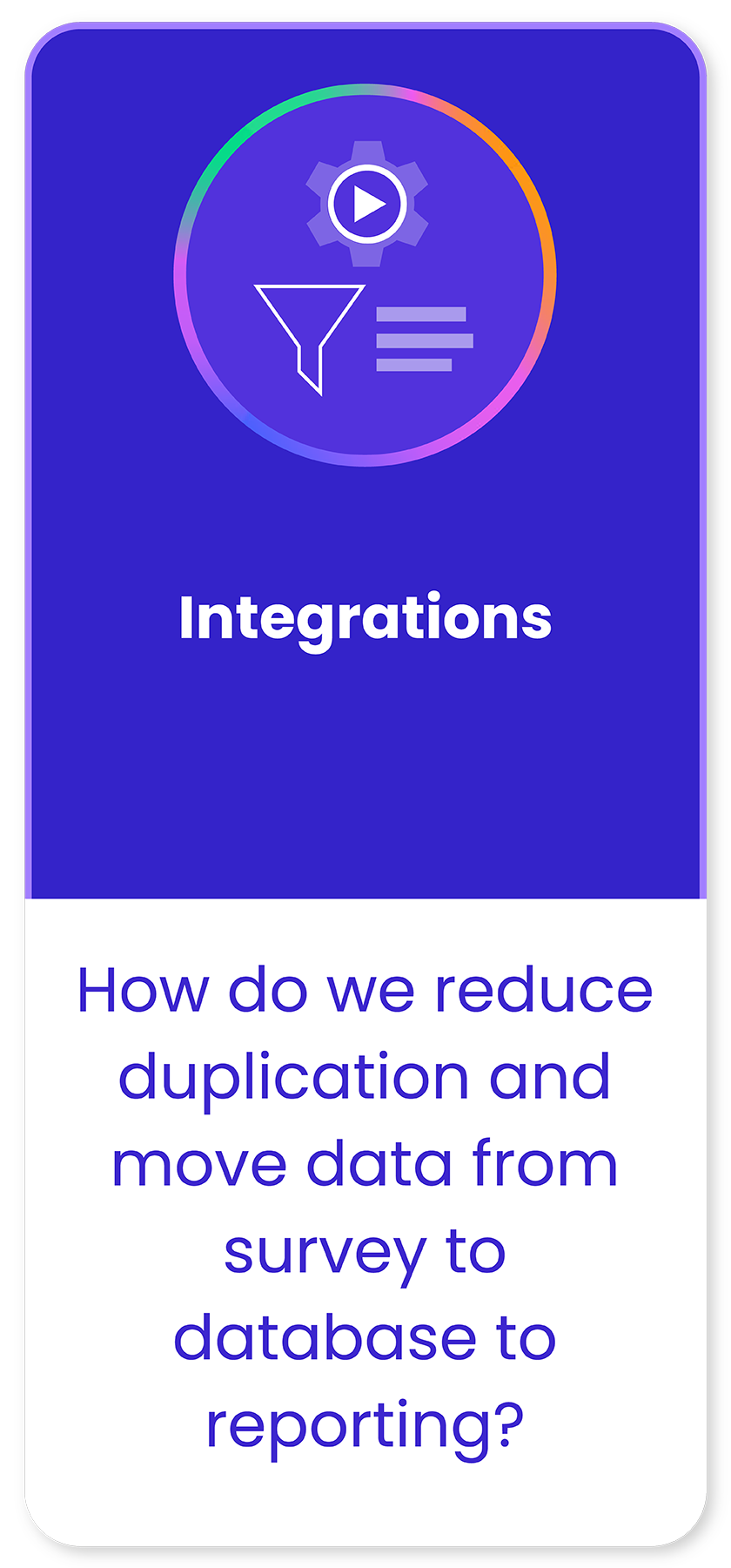
Integrations: Wherever possible, it is ideal to avoid manually handling data and to avoid processes that involve copy-pasting data from one table or spreadsheet to another. This is because these processes tend to introduce human error that can be easily missed, as well as slowing down and using up resources that are better focused on analysing and making use of data than moving data into spreadsheets or visualisations or reports. APIs, and automation tools like N8N, Zapier, Coupler and Sheetgo can help automate processes such as data calculations, standardising and transforming data, creating charts, and so on, and can do it on a cadence that suits your organisational needs (real-time, end of day, monthly, or so on). As with other stages in Phase 2, there is some back and forth: as you create surveys and databases, you may identify new integration and automation needs, and as reports are created, again new integrations may be needed. Often the workflow diagrams created at the end of Phase 1 will help identify integration and automation opportunities, and with AI tools maturing, there may be new ways to integrate and automate data that you can exploit in your data governance systems. At this stage, you are asking "How do we reduce duplication and move data from survey to database to reporting?" That is, where can you automate all aspects of the data journey?
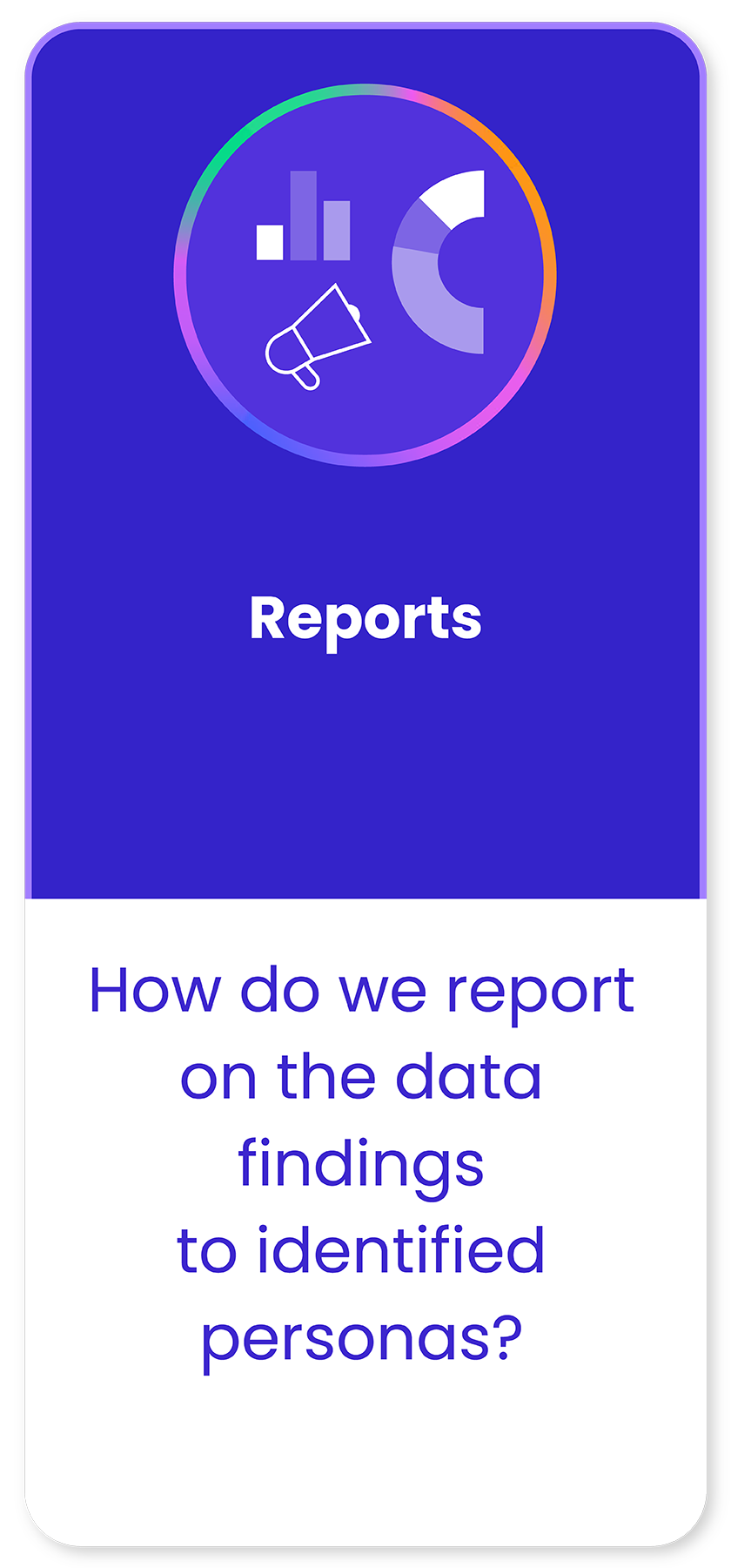
Reports: Drawing on your logic model/theory of change/operational framework, as well as understanding your data ecosystem and personas, will help you brainstorm and plan what reports you need. Your workflow maps will also help you identify what reports you need and what form factors best convey the data to the : PDF or printable summaries, funding and board reports, real-time dashboards, automated social media content assets, and so on. Your integration tooling will help you create your reports automatically, although we find that some analysis is often still needed in terms of a narrative or a discussion of the data findings. At this stage, your goal is to create report templates that meet your workflow and persona needs and ensure your integrations push the data to the reporting forms in a timely manner. Reporting may also be versions of your datasets themselves, especially if you created data sharing agreements when working through your persona and ecosystem maps and workflow tasks. At this stage, you are ensuring you can answer: "How do we report on the data findings to identified personas?"
Phase 1 and 2 of this Data Governance Framework ensures you have asked all of the questions and prepared the policies and procedures that support you to manage data responsibly, ethically, securely and equitably across the data journey. But how can we be sure the data always remains at high quality and is used within any regulatory constraints?
Maintaining quality, security and compliance: Data Assurance and DataOps
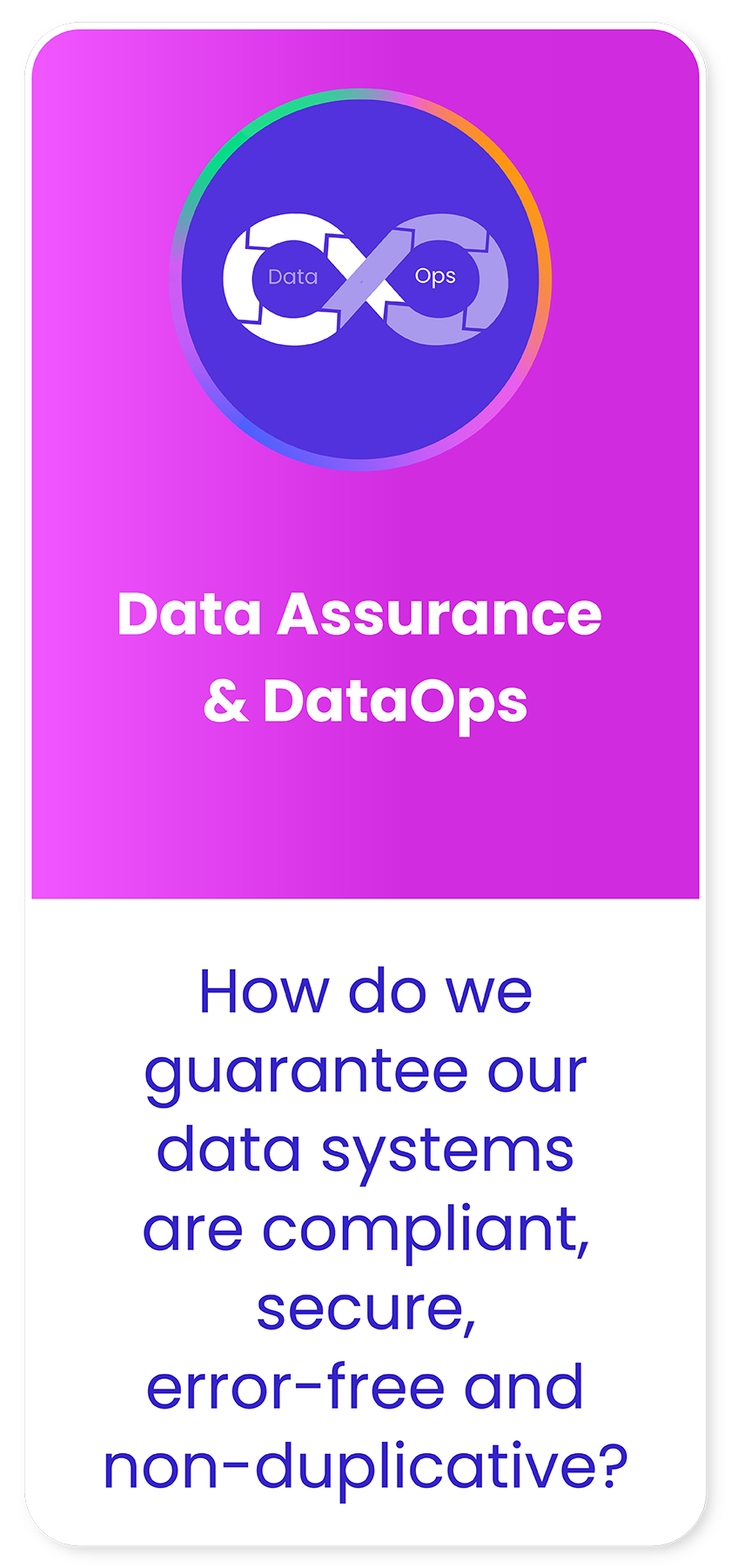
Data Assurance and DataOps processes sit outside Phase 1 and 2 and act as an overall boundary or trust framework that helps you ensure the data side of your organisation is not at risk from data breaches, poor data quality, regulatory non-compliance, or so on. Creating access permission roles, data sharing agreements, and setting licensing provisions for the use of data are all undertaken and monitored in alignment with your data policies. The procedures for this trust framework are not completed at the end: begin your DataOps and Data Assurance process creation when beginning your data governance framework, and revisit and update as each component is fleshed out. Once the data governace framework is then in place, monitoring and regular reviews can be introduced (again drawing, in part, on your chosen data tech stack) to help you answer: "How do we guarantee our data systems are compliant, secure, error-free and non-duplicative?"
Our clients have found our data governance framework to be comprehensive, cohesive, and evolutionary in that for some organisations that have already started their journey, they can advance components by focusing on gaps in their overall model. Others use the framework as a minimum viable approach and when the basics are in place for one program or data area, they revisit the framework to add another department or dataset, and so on, until the data framework covers their whole operations. Others take a Team Topologies approach, where a centralised platform team puts in place the rules, standards, policies and tech stack that allows lines of business to the map the logic flow, personas, data dictionaries and phase 2 elements relevant to their work.
Our model has been built on best practices, industry research, and over 20 years of experience designing and managing data systems for governments, private companies, multilateral organisations and non-profits. We'd love to hear your feedback. Please contact us to discuss these models further, or sign up to our newsletter (in our footer below) to hear more about future articles where we will explore in-depth the evidence behind each step and how to implement each component. We also have online learning courses, in-person training, mentoring support programs, and can offer consultancy projects to support you, but if you follow along our Understand Resource Hub as we build out our data governance content, you will also be able to implement each step of this framework on your own.
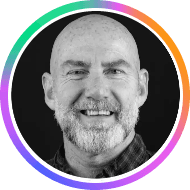
Mark Boyd
DIRECTORmark@platformable.com