Engage
Who benefits from AI in healthcare?
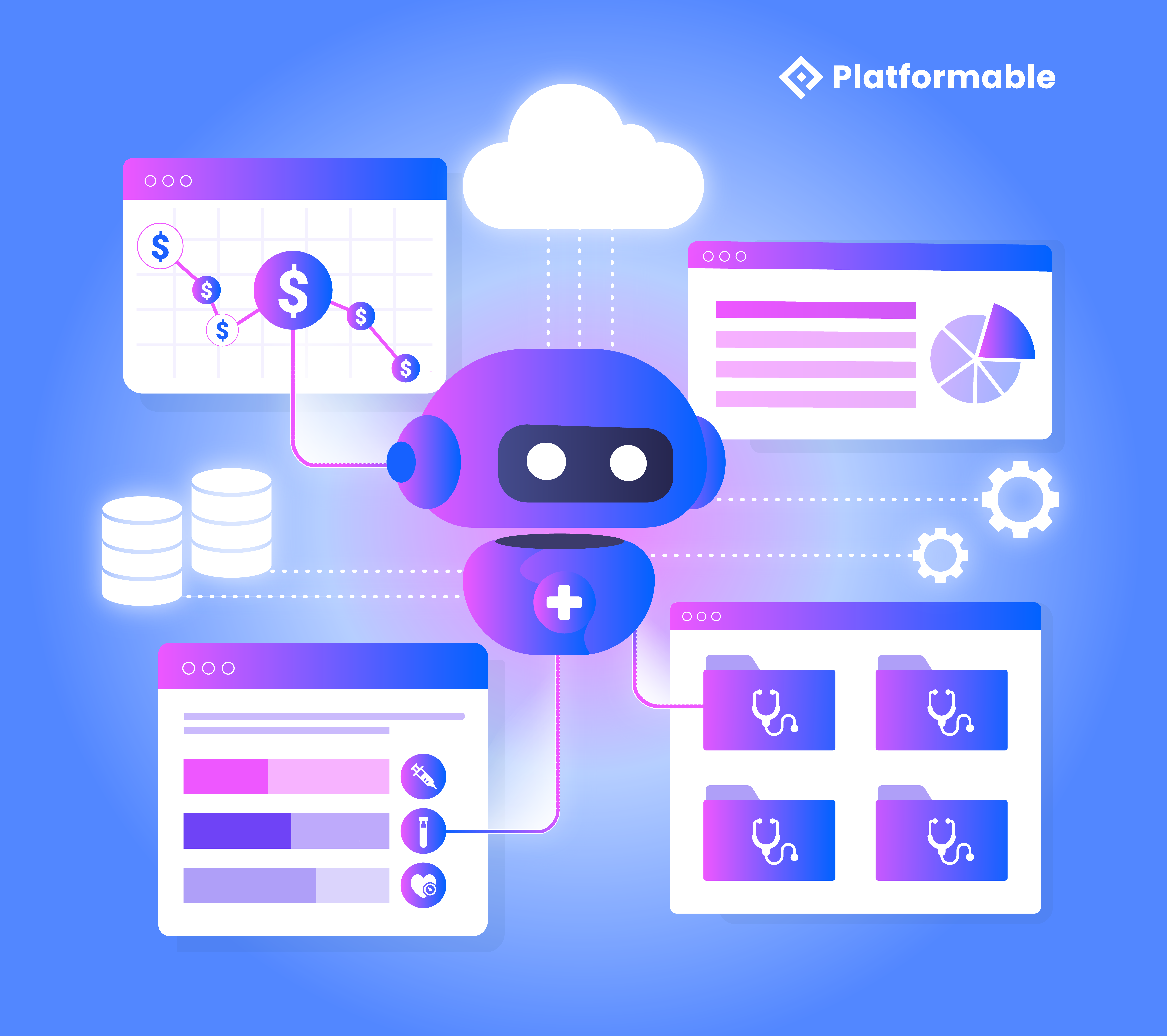
For better or worse, we are building AI in our likeness. Its structure and function “reflects how truly human we are and how we have mirrored that in our tech,” AI ethics educator YK Hong wrote in March 2023. It’s biased, makes wrong predictions, lies and hallucinates, because we do all that. And just as with any tool, AI can be harnessed to help people or harm people, to advance population health or widen health inequality.
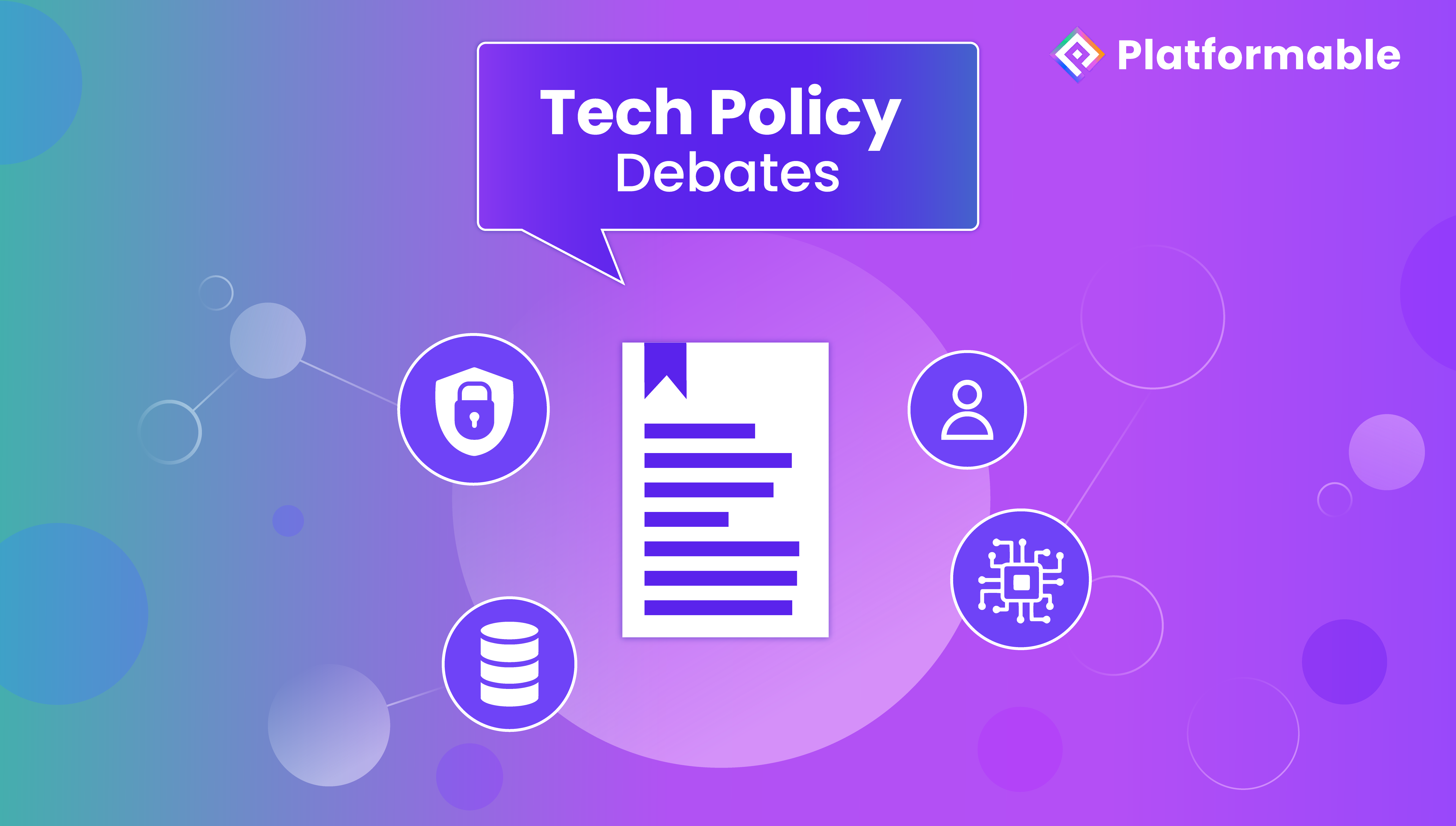
There is no doubt that AI is poised to revolutionize healthcare worldwide. According to a National Bureau of Economic Research study published in 2023, administrative processes account for 25% of healthcare costs. Adopting AI automation and analytics alone could eliminate $200 billion to $360 billion in US healthcare spending within the next five years, along with providing non-financial benefits “such as improved healthcare quality, increased access, better patient experience, and greater clinician satisfaction.”
This last point is especially salient considering that 40% to 60% of healthcare workers report feeling burnout, specifically as a result of having to spend more time on administrative tasks that eat into their time with their patients.
As renowned cardiologist and scientist Dr. Eric Topol notes, AI—specifically automated tools— ultimately return to doctors the “gift of time”. Letting machines take care of tedious and labor-intensive tasks like patient data processing, visit documentation, and screening scores of medical images for routine diagnosis frees physicians up to spend more time actually providing care.
When used as an adjunct — not a replacement to — human care providers, well-coded algorithms can benefit every participant in the equation. “There isn’t any algorithm for empathy,” Dr. Topol said. “This is what we are for — the human connection. We aren’t suddenly going to become more intelligent. But machines are. Our charge is to get more humane.”
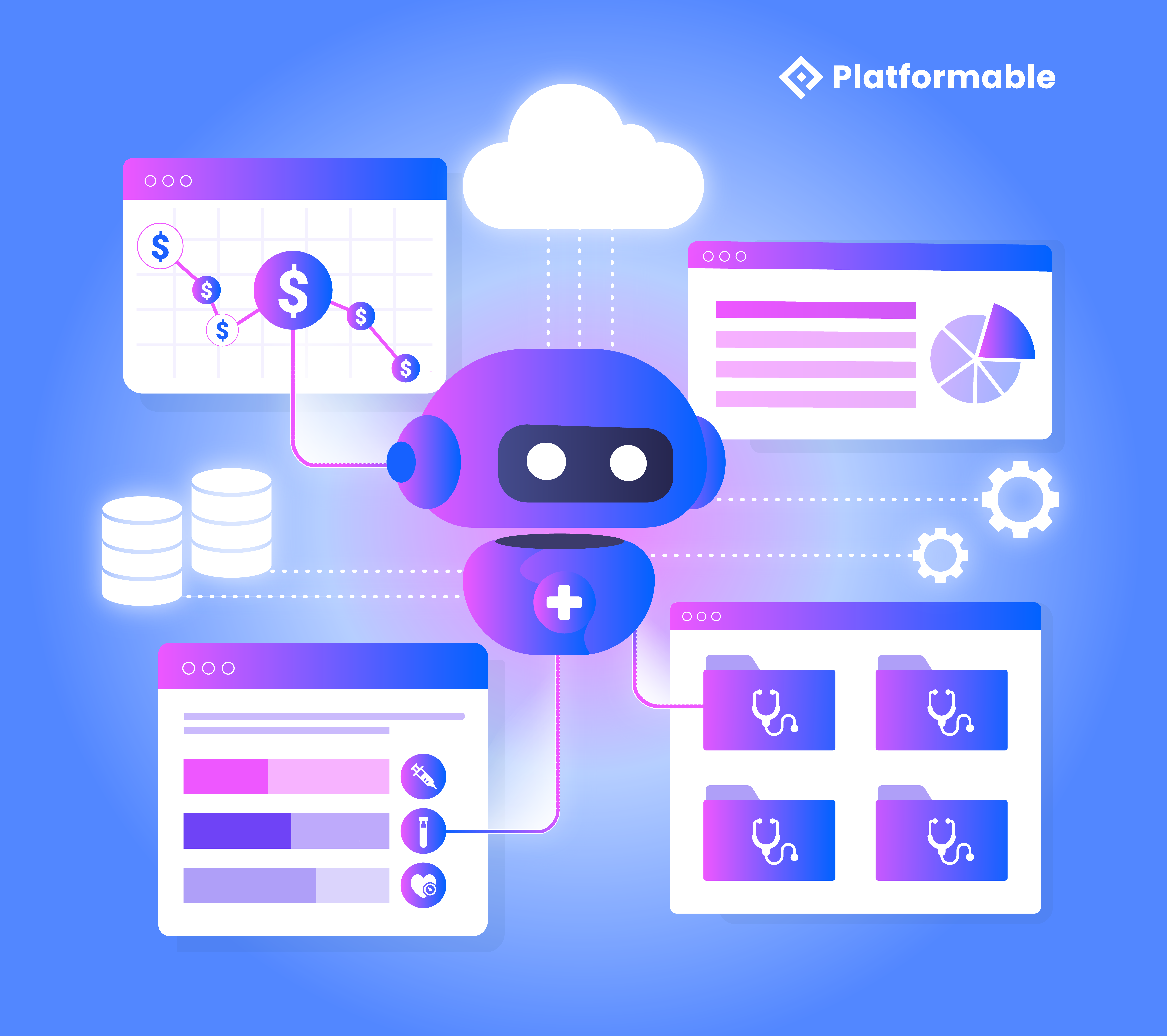
Automating workflows
Allowing providers to become more humane means passing off the rote work to robots. The most easily available automation opportunities are primarily administrative, and include revenue cycle management (RCM), documentation, medication refills, pre-authorization requests, patient onboarding, appointment scheduling and reminders, payment reminders, and the use of AI-based virtual assistants and chatbots.
Regard, a co-pilot AI that helps doctors make diagnoses and draft clinical notes, was found to reduce physician burnout by 50% and documentation time 25%. Because patients forget up to 80% of what they are told by their doctor during a visit, AI tools that record and summarize conversations have been found to boost patient engagement in their own care. Simple appointment reminders via text or phone call significantly raise patient attendance and clinical efficiency. Though seemingly counterintuitive, it’s been shown that workforce automation could actually help ongoing staffing shortages in healthcare.
When well-coded algorithms are employed, workflow automatization without a doubt leads to improved clinical outcomes and lowered costs.
Saved costs = Access to care
Lowered costs brought about by digital health tools have expanded access to healthcare in some of the most resource-constrained parts of the world.
One of the few low and middle income countries in the world to have universal health coverage, Rwanda is a great example of how the adoption of AI automation can dramatically expand access while improving quality of care, cutting wait times, and lowering costs. Rwanda has led the effort of implementing digital health tools, teaming up with AI-powered Babylon Health to provide people access to virtual consultations with doctors and clinical records, and to provide professionals with monitoring and diagnostic tools. As a result, doctors were able to see 80% more patients while underserviced and hard-to-reach populations could receive care virtually rather than walking miles to a clinic.
How this plays out practically is that everyone over the age of 12 is able to consult with clinicians through their mobile phone in minutes, regardless of internet connectivity or phone capability. Patients can digitally book laboratory tests, the results of which go into an Electronic Healthcare Recordd (EHR) accessible to them and their doctor, and receive prescription codes they can pick up from pharmacies nearby. As a result, the country’s community-based health insurance program, Mutuelle de Santé, covers more than 90% of the population and has lowered out-of-pocket spending from 28% to 12%. Incidentally, these digital health tools uniquely prepared Rwanda to manage the COVID-19 pandemic: real-time mapping of the disease’s spread and telemedicine reduced human-to-human contact, while a digital pharmacy system allowed for stable medicine distribution during a time of the highest demand.
Deep learning for diagnostics
True clinical care automation may be nascent, but Large Language Model (LLM) tools are also showing some promise in reducing misdiagnosis, which remains an all-too-common problem. 12 million significant medical diagnostic mistakes are made in the US every year—meaning everyone will experience at least one such error in their lifetime—and that estimate skyrockets on a global scale. Generative AI is already proving to be an especially useful tool for precision medicine as machines scan all the latest available medical research and combine it with patient symptoms to spit out a breadth of possible diagnoses.
For instance, researchers at Rady Children’s Institute in San Diego have been using deep learning algorithms to screen sick children for thousands of genetic anomalies then accurately diagnose them, all within 18 hours of drawing a blood DNA sample. This allows physicians with no rare disease expertise to rapidly have the most up-to-date information for managing critically ill newborns. That same team recently developed a new algorithm capable of scanning 70 million genetic variants (an excerpt 1000 times larger than the previously used archive) in order to instantly identify the ones that make us sick.
Two recent trials for cancer detection in Sweden found AI assisted reading significantly reduced workload and detected more cancers, while a major study published in Nature in September 2023 showed a model trained on 1.6 million retinal images could predict all sorts of diseases, including heart attacks, strokes, and even Parkinson’s and Alzheimer’s. AI programs are evaluating mammograms 30 times faster and with 99% accuracy, radiologists are using algorithms to identify hemorrhages and blood clots in CT scans, facial recognition software is diagnosing and swiftly addressing rare diseases in children.
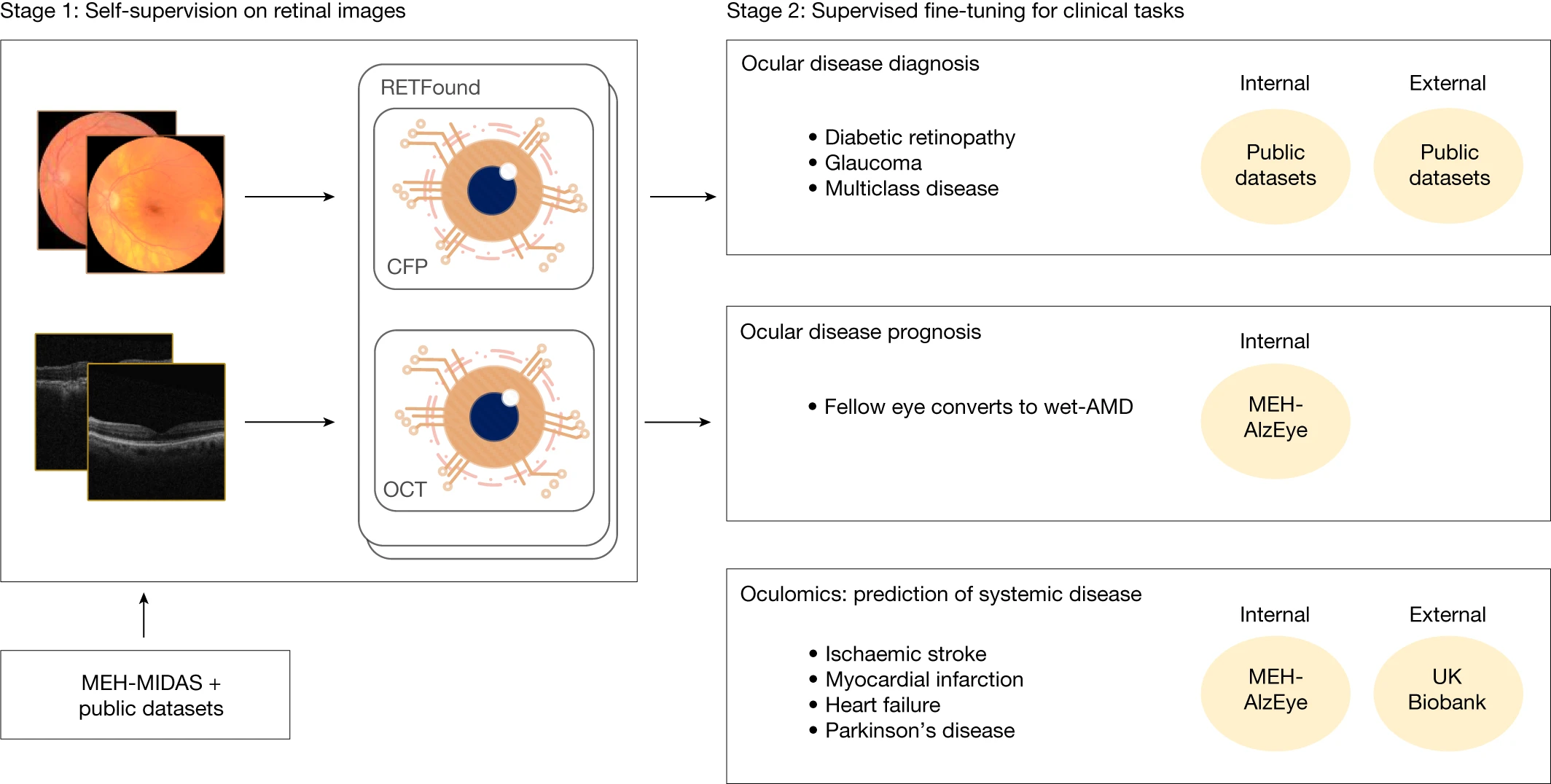
https://www.nature.com/articles/s41586-023-06555-x
Bias and inequality in underlying algorithms
The clearest risk of entrusting AI systems is that just like humans, they can be wrong. Inaccurate diagnoses, missed symptoms, and bad predictions result in patient injury daily, and the widespread adoption of AI systems means an underlying flaw has the potential to harm exponentially more patients than any single provider.
But a more insidious concern than AI’s effectiveness is the potential for bias and inequality in the underlying algorithms. Clinicians statistically provide different levels of care to white people (particularly white men) than they do to people of color and women, and because AI systems mirror our existing health systems, these biases are reflected in the data points being used to train AI tools.
We can already see these systemic issues manifesting in current models: Dr Mahlet Zimeta, in a blog post about colonialism in AI, cites a 2022 Nature Medicine report which estimated “86 percent of genomic research in the world is carried out on genes of people with white European ancestry,” meaning AI models trained on genomic data may not be useful (and may actually be harmful) to the remaining eight billion people on this planet.
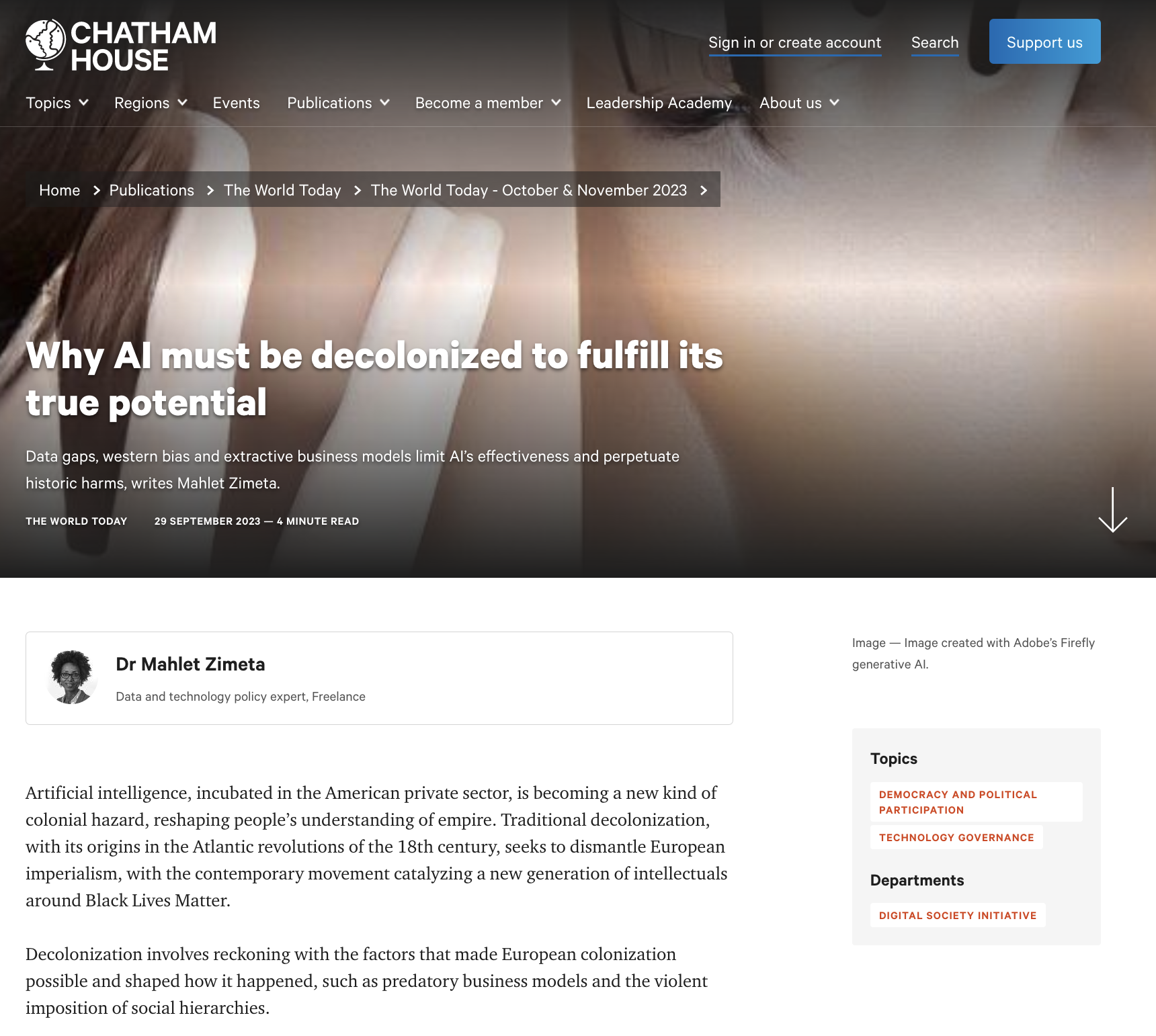
This partiality is by no means an anomaly. There is a well-documented and significant lack of racial diversity in health studies and clinical trials, and it’s been shown that discriminated-against populations are underrepresented in the datasets that train AI systems. The lack of standards surrounding the collection of race and ethnicity data generally means there are a lot of information gaps when it comes to accurate population representation. Recently, the CDC reported that race and ethnicity data were not available “for nearly 40% of people testing positive for COVID-19 or receiving a vaccine.”
As a result, AI systems reflect systemic bias as opposed to biological reality, amplifying patterns of discrimination in the source material that both worsen and reinforce existing social inequalities. Building models off this skewed data will only serve to exacerbate the issue which, as Dr Zimeta points out transforms AI into “a new vector of colonial harm” by upholding “predatory business models'' and violently imposing “social hierarchies.” Until we identify and dismantle the long term effects of imperialism, she argues, “these same factors will affect how AI is developed and deployed.” We need to fix the way we collect and use race data if we want to automate AI in a way that fairly serves all humanity.
A good example of this is a 2019 study published in the journal Science, which found that an algorithm widely used in hospitals to decide which patients needed care was biased against Black people. The algorithm had been trained on healthcare spending to predict healthcare needs, but because Black populations have historically had less resources and access to care, they have spent less on healthcare. As a result, Black patients had to be deemed much sicker than their white counterparts in order for the algorithm to recommend extra care. Similarly, a 2021 study found that patients with darker skin were undertreated for hypoxia because an AI-driven pulse oximeter overestimated their blood oxygen levels. Facial recognition systems have been found to misclassify gender in darker-skinned subjects at a significantly higher rate. Diagnostic tools like temporal thermometers and scalp electrodes have also been found to reflect racial bias.
Machine learning from imbalanced data sets constitutes just part of the problem when it comes to flawed algorithms. It’s worth noting that government institutions and for-profit companies aren’t exactly incentivized to automate access to care and benefits. Author of Automating Inequality Virginia Eubanks writes that programs in the U.S. categorically increase barriers to services because they are “premised on the idea that their first job is diversion,” as has been proven by the outcomes of resource-allocation AI systems deployed across the U.S. over the last decade.
In the mid-2010s, pregnant women and foster children across Colorado and California were improperly denied Medicaid based on algorithms encoded with over 900 incorrect rules and trained on faulty data. Algorithms instituted in Arkansas and Idaho in 2016 made extreme cuts (up to 42%) to in-home care for residents with disabilities and were proven in court to be built off deeply flawed data, but only after the ACLU stepped in. Despite this, “the group that developed the flawed algorithm still creates tools used in healthcare settings in nearly half of U.S. states as well as internationally.”
In the examples above, badly-coded and opaque algorithms (Idaho refused to disclose its AI tool, calling it a trade secret) built off predatory systems that spend hundreds of millions of dollars yearly on deterring patients from seeking care (let alone understanding or challenging the care they are entitled to) ultimately automated a predatory and inefficient process to make it even more predatory and inefficient by allocating fewer resources to patients labeled “less profitable.”
(This feels important to note: processes that are already done well can be automated but automating a broken process will only break it further.)
Privacy and security concerns
Another significant risk for AI is sharing sensitive health data to train and use AI. Building AI tools requires large datasets while using them increases the likelihood of privacy violations, whether through data sharing without informed consent, third-party data breaches, or AI identifying and predicting patient outcomes—non-health ones, and without being asked to.
There are already documented instances of health data being used to deny care to patients based on records, personal choices, and existing medical conditions, often without their knowledge. In July 2023, health insurers UnitedHealthcare and Cigna were sued for denying necessary medical care to elderly patients en masse using a 90% faulty algorithm. The sharing of sensitive health data with third parties like employers and bank and life insurance companies could also have dire implications for consumer safety and privacy.
More worryingly, AI is able to predict private information about patients without the algorithm having received that information. For instance, an AI system might correctly identify that a patient has breast cancer based on genetic, biometric, and lifestyle factors, even if the patient doesn’t know it themselves.
A recent study found that an AI tool trained on medical images had taught itself to identify a patient’s self-reported race “with startling accuracy” despite the image having no relevant patient information connected to it. Researchers don’t know how the machine does it, meaning they don’t know how to fix it.
One big fear is the tech using this information to make race-based healthcare decisions without detection, unintentionally providing worse levels of care to communities of color without intervention and “worse[ning] the already significant health disparities we now see in our healthcare system.” A machine repurposing patient data without consent from either the patient or its handlers highlights the dire need for both transparency and regulation when it comes to designing and deploying AI tools.
How do we fix it?
So how do we ensure we’re using AI in a way that helps rather than harms? We start with awareness. As YK Hong put it, “just as we have criteria when interacting with humans, for example, stranger danger or getting to know someone you’ve just met before trusting them, don’t abandon those skills when interacting with AI.”
Thinking purposefully about mitigating the risks posed by AI-driven bias and privacy concerns starts with effective data governance. Using broad, high-quality data sets in a way that prioritizes patient privacy and maintains efficient interoperability can become expensive, but there are steps to be taken that don’t cost a lot of money.
One approach advocated by many strategists and analysts recommends first creating an organizational infrastructure aimed at fostering equity, starting with establishing AI use boundaries, setting up an oversight committee, and defining risk controls, and ending with applying governance, risk, and legal frameworks that besides bias account for data security and regulatory compliance.
This includes using AI as a partner tool rather than a human replacement, to combat inaccuracy and bias but also because a machine can never provide better “care” than a human doctor. As it currently stands, hospitals using AI to automate administrative tasks require doctors and nurses to review the AI-generated documents before they’re added to patient records. It also means investing in people who deal specifically in including equity at the forefront of operations.
Once an AI system has been deployed, continual monitoring and cooperation between various involved players (AI developers, healthcare providers, regulatory bodies) will be key in aligning with do-no-harm AI philosophy and management.
Ultimately, AI automation through machine learning and deep learning removes human error from redundant, manual tasks, leading to more efficient and accurate patient care, happier healthcare workers, and wider access, while saving everyone time and money. But it's no panacea to the problems plaguing existing systems across the world. Organizations and companies need to first and foremost implement tools that automate processes they already do well, and do it with an eye towards helping and protecting humankind.
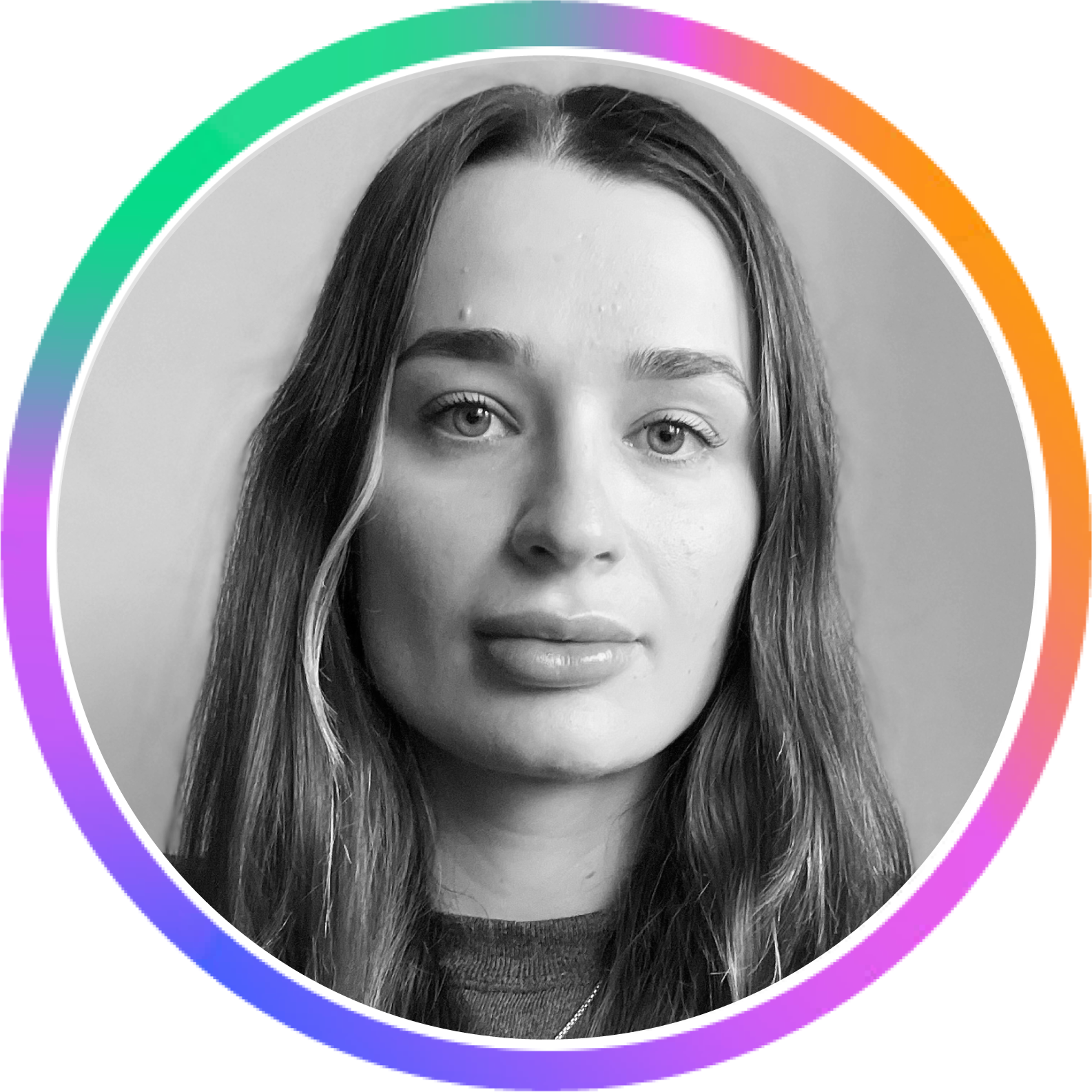
Mariam Sharia
TECH POLICY WRITERaccounts@platformable.com